Decoding Hit Discovery: From DEL to Virtual Screens
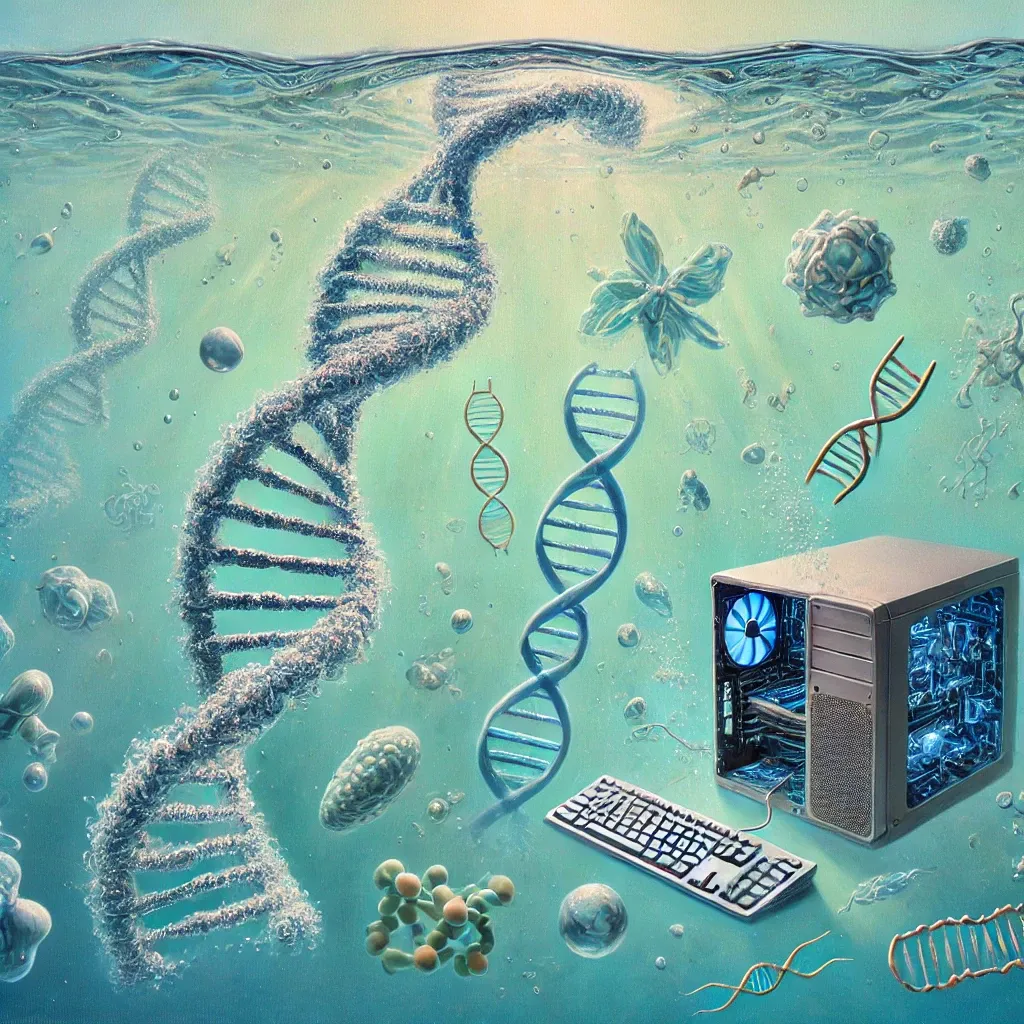
You've identified a biomolecule you want to target (for a refresher on target identification, see our previous article), and now you're ready to launch a hit identification campaign. In this article, we'll focus primarily on small molecule hits for protein targets, while addressing non-small molecule hits and other biomolecules in future pieces.
Modern small molecule hit discovery, as conducted in major pharmaceutical and biotech companies, typically falls into four main categories:
1. High-throughput screening (HTS)
2. Fragment screening
3. DNA-encoded library (DEL) screening
4. Virtual screening
This article assumes familiarity with these techniques and instead concentrates on the decision-making process when selecting and implementing a screening method.
It's important to note that these methods can be costly. For instance, DNA-encoded library and HTS campaigns can range from $100,000 to $500,000 each. Given this significant investment, careful consideration of each method is crucial before embarking on a screening campaign. Let's examine a few scenarios in detail to guide your decision-making process.
Fragment Screening
When pure protein expression has been previously reported, either internally or in literature, fragment screening and/or DNA-encoded library (DEL) screening become the methods of choice.
I have a special affinity for Fragment-Based Drug Discovery (FBDD), as it was the focus of my PhD research. My doctoral lab collaborated with Astex Pharmaceuticals, a pioneering company in FBDD, providing me with the invaluable experience of interacting with world experts in the field.
Fragment screening is particularly advantageous when the target protein has been previously crystallised. While crystallisation isn't strictly necessary for fragment screening, hit optimisation becomes significantly more challenging without structural insights. If you opt for fragment screening, you'll need a robust primary biophysical assay. Surface Plasmon Resonance (SPR) is typically preferred, although Nuclear Magnetic Resonance (NMR), Isothermal Titration Calorimetry (ITC), and crystallography are also viable options. SPR is often the primary screening method due to its relatively straightforward assay development and higher throughput compared to other biophysical methods. Fragment libraries usually contain 1,000-5,000 compounds. While this is considerably smaller than HTS or DEL libraries, the lower molecular weight of fragments allows for broader coverage of chemical space with fewer compounds. For those considering building a fragment library, I recommend reading this excellent article by my former colleagues at AstraZeneca.
After the primary screen, hits are typically confirmed using an orthogonal biophysical assay such as NMR or ITC. Due to their low molecular weight, fragment hits require careful consideration of binding efficiency. Metrics like Ligand Efficiency (LE) help prioritize the most effective fragment hits, ensuring that every atom contributes to binding. For those interested in staying updated on fragment drug discovery, I highly recommend following this informative blog.
DEL screening
DEL screening is as an excellent option when purified protein is available. One of its key advantages is the minimal protein requirement - typically no more than 2-3 mg - making it particularly valuable when protein expression is challenging. However, protein quality is crucial for DEL screening. The protein must be highly pure, in its functional form, and stable (non-aggregating) under screening buffer conditions.
Budget permitting, DEL screening can be conducted in parallel with fragment screening. This approach is beneficial as insights from one method often complement the other. For instance, knowledge about protein immobilisation tags and buffer choices from SPR in fragment screening can be directly applied to DEL screening.
In theory, DEL screening provides access to billions of compounds. However, this vast number can be somewhat misleading in terms of chemical diversity. Each library typically contains a single scaffold (although some chemistries allow for multiple scaffolds), which can only explore limited parts of the 3D chemical space. Thus, a million-member library might not represent as much chemical diversity as the number suggests. Nevertheless, through a collection of libraries, DEL screening can access a wide range of 3D chemical spaces.
A major advantage of DEL screening over traditional High-Throughput Screening (HTS) is its compact nature. Billions of compounds fit into a microliter-sized plastic tube, whereas HTS requires massive infrastructure to house millions of compounds and complex robotic systems for plating and assaying.
Given these advantages, one might wonder why DEL screening isn't used for everything. There are limitations. For instance, it's not ideal for DNA-binding proteins like transcription factors, although careful experimental design (such as blocking the DNA binding site with excess consensus binding sequences) can sometimes overcome this. Most importantly, impure or aggregated protein is an absolute dealbreaker for DEL screens - it's not even worth attempting in such cases.
While DEL screening is rapid once purified protein is available, it involves a crucial post-screening phase. This phase requires the synthesis of compounds identified through analysis of DNA sequences enriched in the presence of your target. The off-DNA synthesis typically takes 4-6 months.
The entire DEL screening process can span 9-12 months, encompassing:
· Protein expression
· Quality control to ensure protein purity and functionality
· Screening, sequencing and data analysis
· Off-DNA synthesis
This extended timeline could be a significant drawback for teams aiming for quick progress. However, there's a strategy to accelerate the process: leveraging the vast data generated from the screen to build machine learning (ML) models. These models can be used for ligand-based virtual screening, predicting binders from internal or commercial suppliers. This approach can yield hits well before off-DNA synthesis begins. For those considering DEL screens, the paper from X-Chem and Google is an essential read. It details valuable insights into developing graph-based ML models using DEL data for binder predictions.
Lastly, DEL has exciting potential applications in PROTACs (Proteolysis Targeting Chimeras) and other platforms that requires chimeric compounds. Its unique structure, with compounds attached to DNA, opens possibilities for developing PROTACs or other chimeric compounds. The DNA attachment point can serve as a handle for building these complex molecules as you can be confident that during a binding event, a linker built from the handle will be tolerated. Moreover, this feature can also be exploited to improve properties of compounds such as solubility.
Virtual Screening
Let's shift our focus to virtual screening, a method that has gained significant traction in recent years. Several companies now offer multi-billion compound virtual libraries, with these numbers expanding by billions annually. Enamine's fantastic team popularised these virtual libraries, with many companies reporting over 80% success rates in successful delivery of ordered virtual compounds from Enamine- a testament to Enamine's synthesis and cheminformatics expertise.
Virtual screening can be broadly categorised into two main approaches:
1. Structure-based:
If you have a crystal structure of your protein and are confident about the binding pocket, you can dock billions of compounds. OpenEye's gigaDOCK offers an excellent solution for docking billion-member libraries. However, a major caveat is the unreliability of docking scores, which may cause you to overlook genuine hits. To further refine the list, physicochemical filters such as logP and the number of H-bond donors or acceptors are typically applied.
2. Ligand-based:
When a known ligand binds to the protein of interest, it can be used to find similar compounds from virtual collections. Similarity metrics can be 2D or 3D-based. In 3D methods, you use either the known conformer of the ligand or 10-20 calculated conformers to find compounds matching their shape and electrostatics. OpenEye's fastROCS excels at ligand-based virtual screening.
In the ideal scenario, where a crystal structure of a potent ligand bound to the protein is available, you can combine ligand and structure-based methods. Typically, you'd start with the ligand's conformer from the crystal structure to find compounds with similar shape and electrostatics from commercial virtual libraries. After applying 3D-based similarity metrics and physicochemical filters, you'll have a significantly reduced set of compounds. These can then be docked into the binding pocket, retaining only those that dock as desired. If the number is reduced to few hundred, you can apply physics-based calculations such as absolute binding free energies or molecular dynamics-based MMPBSA calculations to triage further.
You might be wondering what about structure-based methods using AI-generated protein conformations from AlphaFold or RosettaFold. While feasible, it's crucial to remember that proteins are dynamic entities. Various domains can move significantly in the presence of a ligand - a complexity that AI algorithms predicting protein structures alone cannot model. One alternative is to rely on physics-based simulation methods to explore all potential protein conformations starting from the conformation generated by AI based methods. In theory, simulating the movement of every atom for several seconds would sample all possible conformations. However, this is often impractical without a supercomputer, and even then, simulations can get trapped in local minima, failing to sample all possibilities. Fortunately, methods exist that can sample many conformational spaces in a short time, such as steered MD, REST MD, and metadynamics. After MD simulations, you can cluster protein conformations and use centroids of each cluster to represent various protein states. You can then either use ensemble methods or treat each conformation as a distinct protein. I generally prefer treating each conformation separately, as ensemble-based approaches might penalize genuine hits that bind to only one specific conformation. The main drawback is that centroids might miss the actual conformation required for binding. Unfortunately, there's no practical workaround, as performing virtual screening against thousands of individual conformations from MD simulations would be computationally and practically prohibitive.
Recent developments include AI-based methods (mainly diffusion-based) that attempt to model proteins and small molecules together. While I haven't yet seen a practical example where this outperformed traditional docking methods in a drug discovery campaign, it's still early days. I'm confident these methods will improve over time.
As the field of virtual screening continues to evolve, integrating AI, protein dynamics, and traditional methods, we can expect more sophisticated and accurate approaches to emerge, potentially revolutionising the hit identification processes.
High throughput screen
Let's conclude with a brief discussion on High-Throughput Screening (HTS). Though it's been a staple in drug discovery for decades, HTS remains one of the most widely used methods in hit-finding campaigns. The primary advantage of HTS lies in its modularity and versatility. It allows researchers to dig deeper into binding mechanisms through a variety of assay options, from FRET and AlphaLISA to CETSA and various cell-based screening. Importantly, HTS doesn't always require purified protein, making it an obvious choice when protein purification is a bottleneck, provided you can develop a functional assay. One might argue that purified protein will eventually be necessary for downstream biophysical assays or structure-based drug discovery. Nevertheless, for organisations with libraries of several hundred thousand to a few million compounds, HTS remains highly appealing. Unlike DNA-Encoded Library (DEL) screening, HTS takes longer to set up. However, it offers the advantage of immediate compound availability once hits are identified.
Data analysis for hit triage is crucial to distinguish genuine hits from noise. Careful clustering methods and historical binding data can help identify hit clusters with structure-activity relationships (SAR). Due to the scale of robotic plating, minor issues can sometimes cause true hits to show lower values in the primary assay. To mitigate this, it's common practice to screen twice in the primary assay, with the second round focusing on compounds selected based on data analysis and clustering output of the first-round results. This approach allows for the "rescue" of potentially valuable hits that might have been missed initially.
The success of HTS hinges on thoughtful consideration of assay design and data analysis pipeline as you progress from primary to secondary screening and final confirmation. A critical question to consider is whether your assay is designed to identify hits with the desired mechanism of action.
Affinity Selection Mass Spectrometry (ASMS) deserves a mention in the methods of hit finding. It is a type of High-Throughput Screening that shares similarities with DNA-Encoded Library (DEL) screening in its reliance on direct binding of hits to the resin bound protein. However, ASMS uses mass spectrometry for hit deconvolution rather than DNA sequencing.
ASMS requires carefully curated libraries to ensure each compound is detectable by mass spectrometry. It also demands higher protein quantities compared to DEL screening. Given these constraints, ASMS may not offer significant advantages over DEL if the chemical spaces covered by both libraries are similar. However, ASMS can be particularly useful when its library explores different chemical space than DEL, or when working with proteins incompatible with DEL screening, such as DNA-binding proteins.
Outsourcing Screening Services
For companies or academic labs lacking in-house screening facilities, several companies offer specialised services. While this list is not exhaustive and reflects my personal experience, it provides a starting point for those seeking screening partners. Remember, capabilities are continually evolving, so it's worth exploring beyond these suggestions. If your company is not in the list and you would like to be mentioned then please contact us.
1. DEL Screening:
2. Virtual Screening:
- Tools: OpenEye, Schrödinger, BioSolveIT
- Virtual Libraries: Enamine
3. HTS and Fragment Screening:
I hope this article has provided a valuable overview of hit discovery methods. While we've covered significant ground, there are still many areas left unexplored, such as screening non-small molecules like peptides, or targeting other biomolecules like RNA or DNA. These topics deserve their own dedicated discussions, which we'll address in future articles.
We plan to delve deeper into each screening method described here. To stay informed about these upcoming, more detailed explorations, please consider subscribing.
We value your input and engagement. If you have any questions, or if there are specific hit-finding strategies, methods, analyses, or assays you'd like us to cover in detail, please don't hesitate to reach out. Your feedback and suggestions will help shape our future content, ensuring we address the topics most relevant to our readers.